Enough is too much?
The main benefit of the traditional clinical trial is that it provides causal knowledge: a successful trial tells us that a drug causes the patient to get better. The modern alternative is to search very large patient data bases for interesting associations. This holds both enormous promises and enormous perils - promises because we can test for lots of correlations cheaply and on interesting populations; perils because we run a huge chance of 'overfitting' the data, and because we never know whether the association we found is causal. We might see in our data base that people who take anti depressants and statins tend to develop diabetes; but is the onset of diabetes actually caused by the connection, or do people who take statins and are in the early stages of diabetes tend to get depressed? If one finds associations like this, one has to run an expensive clinical trial after all to get certainty about the causal direction. Yet, there exist a lot of recently developed - but not yet widely used - tools that allow one to get a fair amount of causal information from the database directly. While these never give certainty, they do point towards 'hopeful' and 'nearly hopeless' causal conjectures, so one can use them to decide what clinical trials are really worth trying. The goal of the project is to explore and refine such tools for medical applications.
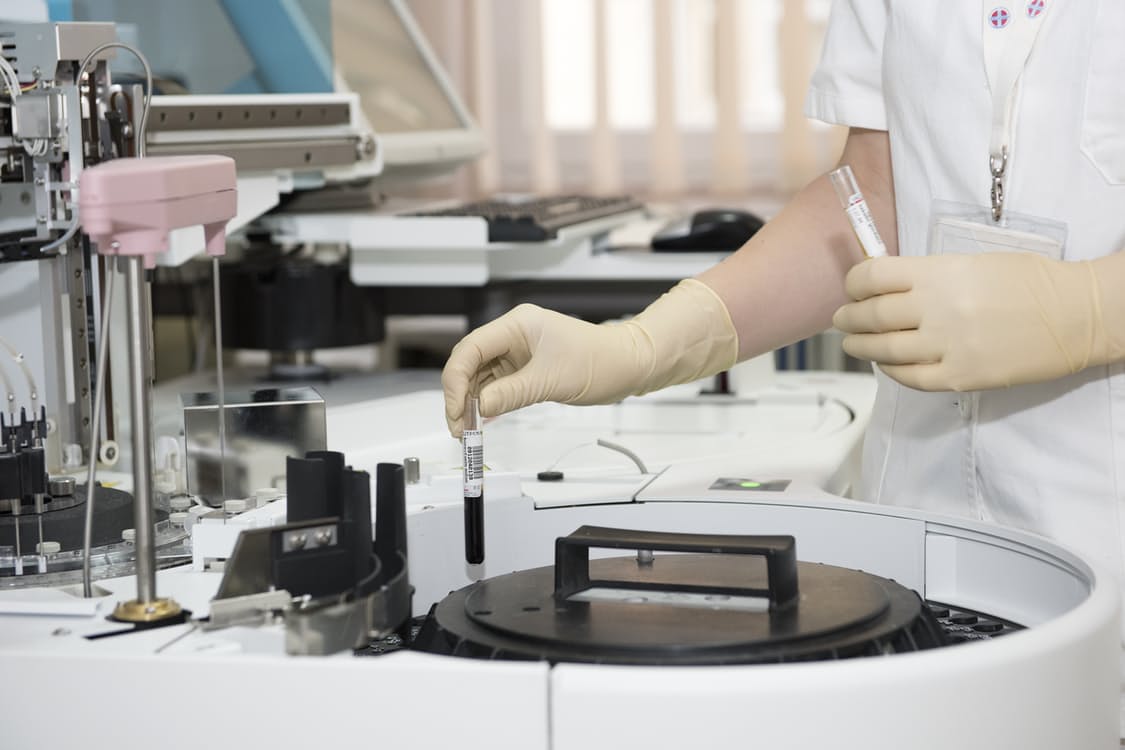