Efficient Deep Learning for MRI
How to learn useful features for classifiers when only few examples are available? In a paper recently accepted for publication in NeuroImage the authors T. Adel, T. Cohen, M. Caan and M. Welling present a new method to use deep learning for analyzing brain MRI images. The technology first processes an MRI image using a so called "3D scattering transform", after which a standard classifier such as a support vector machine is trained. The scattering transform is a fixed transform inspired by convolutional neural networks to extract useful features from the image. This method has the distinct advantage that it works very well when the classifier is trained on small datasets. The model is also extended to a semi-supervised setting where both labeled and unlabeled data are used to further improve data efficiency. The method is shown to improve the state of the art on a number of benchmarks to detect early signs of dementia. For instance, using a subset of the ADNI dataset it is shown that 82.7% accuracy can be achieved in predicting whether a patient with "mild cognitive impairment" (MCI) will convert over a three year period to Alzheimer's disease (progressive MCI) or not (stable MCI). This represents an 8% points improvement over the previous state of the art on this task (74.7%). We anticipate that this method represents a very reliable processing pipeline for analyzing a wide range of medical images in a regime where datasets are not very large.
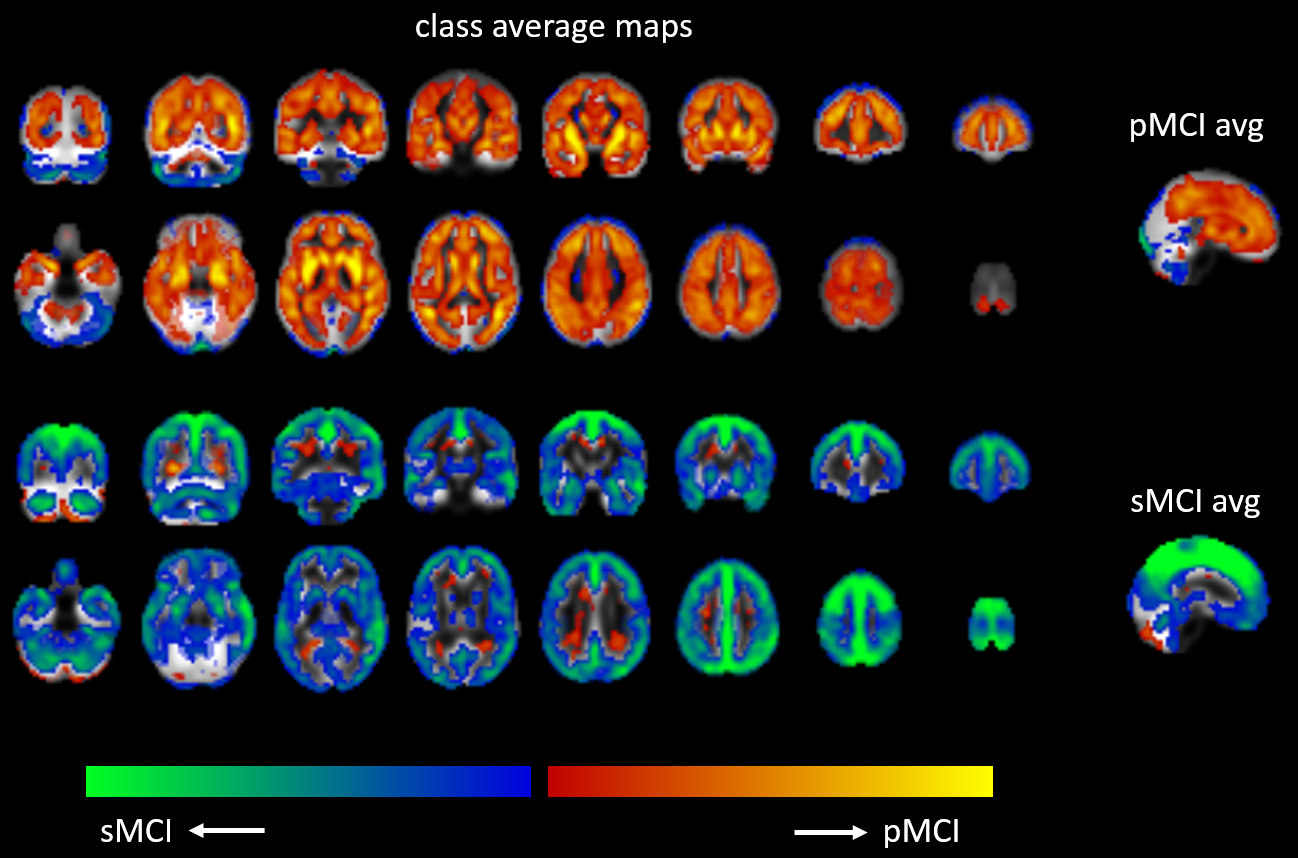
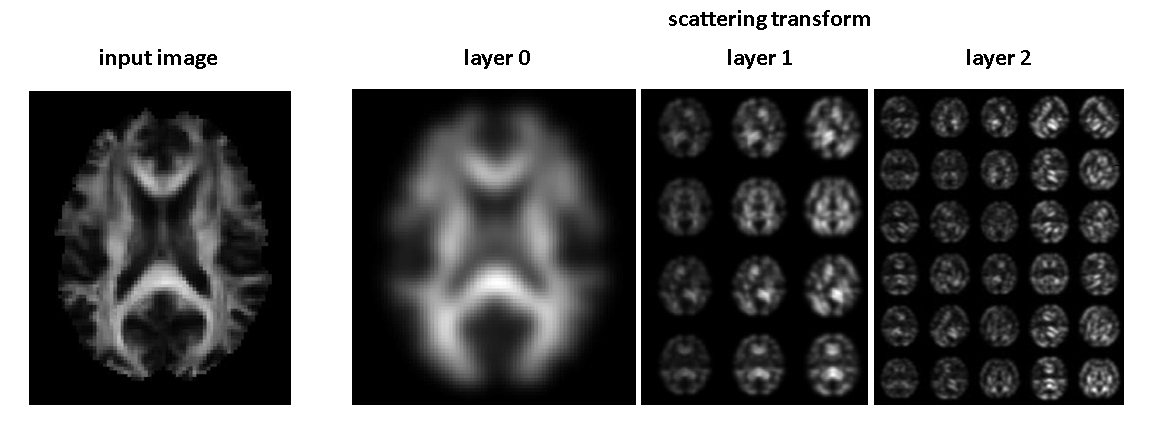